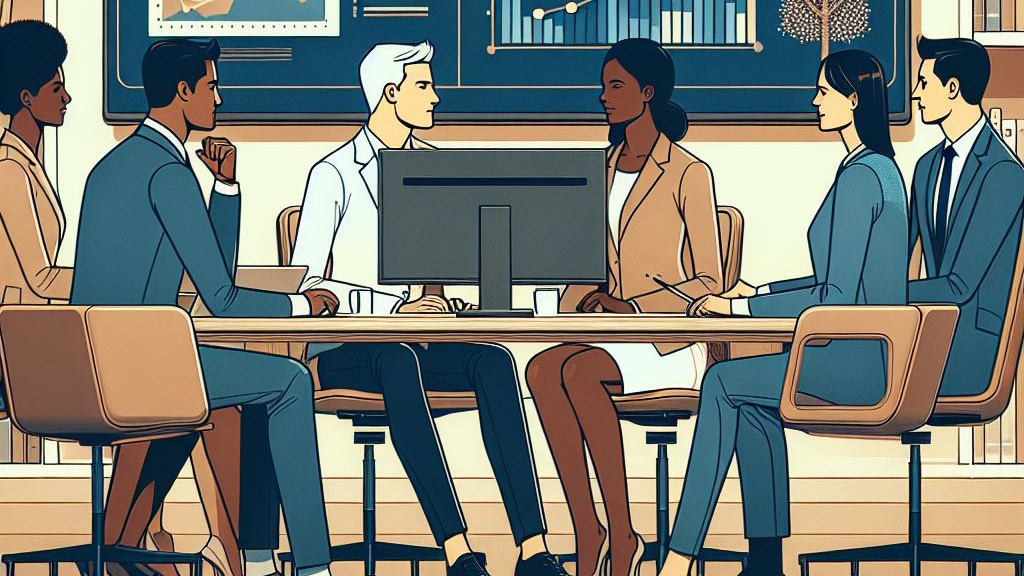
Understanding the Evaluation of Large Language Models: A Guide for State and Local Government Agencies
In the rapidly evolving landscape of artificial intelligence, state and local government agencies are increasingly exploring the potential of large language models (LLMs) to enhance their operations. However, evaluating these models can be challenging, especially when comparing offerings from different providers like Google, AWS, and Microsoft. This blog post aims to provide a clear framework for understanding how to properly evaluate LLM solutions and ensure that results are not skewed by supplementary processes such as Retrieval-Augmented Generation (RAG) or embedding models.
1. The Basics of Large Language Models
Large language models are AI systems trained on vast amounts of text data to understand and generate human-like language. They can perform a variety of tasks, from answering questions to generating content. However, the effectiveness of an LLM depends on several factors, including the quality of the training data, the architecture of the model, and the specific use case it is applied to. When evaluating LLMs, it is crucial to understand these foundational elements to make informed decisions.
2. The Role of Retrieval-Augmented Generation (RAG)
RAG is a technique that enhances the capabilities of LLMs by integrating external knowledge sources. This process involves retrieving relevant information from a database or the internet and using it to generate more accurate and contextually relevant responses. While RAG can significantly improve the performance of an LLM, it can also introduce variability in the results. Therefore, when comparing LLM solutions, it is essential to consider whether and how RAG is being used, as it can impact the perceived quality and consistency of the model’s outputs.
3. The Importance of Embedding Models
Embedding models play a critical role in how LLMs understand and process language. These models convert words and phrases into numerical vectors that capture their meanings and relationships. Different providers may use different embedding techniques, which can affect the performance of their LLMs. When evaluating LLM solutions, it is important to understand the embedding models being used and how they influence the results. This understanding can help ensure that comparisons between different LLMs are fair and based on the underlying technology rather than supplementary processes.
4. Evaluating LLM Solutions: Key Considerations
To properly evaluate LLM solutions, agencies should consider several key factors:
- Accuracy and Relevance: Assess the model’s ability to generate accurate and contextually relevant responses.
- Consistency: Evaluate the consistency of the model’s outputs across different queries and use cases.
- Transparency: Understand the methodologies and technologies used by the provider, including RAG and embedding models.
- Scalability: Consider the model’s ability to scale and handle increasing amounts of data and queries.
- Cost: Evaluate the cost-effectiveness of the solution in relation to its performance and benefits.
5. Data Privacy and Security
One of the most critical aspects of evaluating LLMs for government use is data privacy and security. Agencies must ensure that the LLM provider complies with relevant regulations and standards, such as GDPR or CCPA. Additionally, understanding how data is stored, processed, and protected is essential to prevent unauthorized access and data breaches.
6. Customization and Fine-Tuning
The ability to customize and fine-tune an LLM to specific needs can significantly impact its effectiveness. Some providers offer more flexibility in this regard, allowing agencies to adapt the model to their unique requirements. Evaluating the ease and extent of customization options is crucial for ensuring the LLM can meet specific operational needs.
7. Performance Metrics and Benchmarks
When comparing LLM solutions, it is helpful to use standardized performance metrics and benchmarks. These can include measures such as accuracy, response time, and resource utilization. By using consistent metrics, agencies can make more objective comparisons between different LLM offerings.
8. Ethical Considerations
Ethical considerations are increasingly important in the deployment of AI technologies. Agencies should evaluate how LLM providers address issues such as bias, fairness, and transparency. Understanding the ethical frameworks and practices of the provider can help ensure that the LLM is used responsibly and equitably.
9. Vendor Reputation and Track Record
The reputation and track record of the LLM provider can provide valuable insights into the reliability and quality of their solutions. Researching the provider’s history, customer reviews, and case studies can help agencies gauge the provider’s expertise and commitment to delivering high-quality AI solutions.
10. Future-Proofing and Innovation
AI technology is constantly evolving, and it is important to choose an LLM provider that is committed to innovation and continuous improvement. Evaluating the provider’s roadmap, investment in research and development, and ability to adapt to emerging trends can help ensure that the chosen solution remains relevant and effective in the long term.
11. Community and Ecosystem
The strength of the community and ecosystem surrounding an LLM can also impact its effectiveness. Providers with active developer communities, extensive third-party integrations, and robust ecosystems can offer additional resources and support that enhance the overall value of the solution.
12. Real-World Use Cases and Success Stories
Examining real-world use cases and success stories can provide practical insights into how an LLM solution performs in similar contexts. Agencies should look for case studies and testimonials from other government entities or organizations with similar needs to understand the potential benefits and challenges of the solution.
13. Pilot Programs and Trials
Before committing to a full-scale implementation, agencies can benefit from pilot programs and trials. These allow for hands-on evaluation of the LLM solution in a controlled environment, providing valuable data on its performance, usability, and integration capabilities.
14. Feedback and Continuous Improvement
Finally, it is important to establish mechanisms for ongoing feedback and continuous improvement. Regularly assessing the performance of the LLM solution and gathering feedback from users can help identify areas for enhancement and ensure that the solution continues to meet evolving needs.
Conclusion
Evaluating large language models is a complex process that requires careful consideration of multiple factors. By understanding the role of RAG, embedding models, and other critical variables, state and local government agencies can make informed decisions that align with their specific needs and objectives. This comprehensive approach will help ensure that the chosen LLM solution delivers maximum value, enhancing the efficiency and effectiveness of government operations.